You’ve been trying to figure out quantitative content analysis, but no matter where you look, all you find are books, papers, and information on qualitative content analysis.
Help is on the way.
Quantitative content analysis often takes a backseat to its qualitative counterpart, receiving only a brief mention in methodology books. However, if this is the method you want to apply, you require more guidance. And that’s exactly what you’ll get here.
In this article, you will learn how to conduct a quantitative content analysis in seven steps and understand the key differences from qualitative content analysis.
Quantitative vs. Qualitative Content Analysis: The Key Differences
Quantitative content analysis traces back primarily to a methodology book by social psychologist Bernard Berelson. He defined content analysis as a “research technique for the objective, systematic, and quantitative description of the manifest content of communication” (Berelson, 1952, p. 489).
Note: This definition applies to content analysis in general, not just the quantitative approach. Naturally, this sparks debate, as the very term “quantitative” can provoke strong reactions from advocates of the qualitative research paradigm.
The subject matter of content analysis—whether qualitative or quantitative—is always somehow qualitative in nature. That is because content analysis helps us evaluate qualitative data sources, such as newspaper articles, films, social media posts, or documents. But the method itself is heavily informed by the quantitative paradigm, as its name suggests.
Quantitative content analysis systematically converts qualitative material into quantifiable data by applying structured coding schemes and statistical methods. We’ll explore how that works shortly.
Both quantitative and qualitative content analysis aim to systematically and objectively evaluate content. However, a key distinction is that the quantitative approach allows for greater intersubjective traceability, as it follows a structured and replicable coding process.
While qualitative content analysis relies more on the researcher’s judgment and interpretative creativity, quantitative content analysis follows a strict set of rules. It is designed to test theories by verifying hypotheses rather than generating new ones.
Let’s look at the 7 steps of applying quantitative content analysis.
Step #1: Theoretical Preparation for Quantitative Content Analysis
As with any research project within the quantitative paradigm, engaging with existing theories is crucial. Start by defining your research problem—what exactly do you want to investigate?
Ideally, your problem should focus on the relationship between variables that you can examine through content analysis. For example, you might study “news framing” related to climate change and the “emotions” in social media discussions.
Before conducting your quantitative content analysis, formulate hypotheses—testable assumptions about the relationships between variables. A strong hypothesis clearly defines the dependent and independent variables and ensures that the coding categories reflect these constructs. For example, in a study on climate change news framing, you might hypothesize that news articles from government-funded media use the ‘scientific consensus’ frame more often than private news outlets. Another example: “Climate change news framing (independent variable) influences the emotional responses of social media users (dependent variable).”
For a deeper dive into hypothesis formulation, check out my dedicated tutorial on the topic.
Step #2: Sampling
Now, you need to determine your sample. Suppose you want to analyze how climate change is framed in social media. Your sample could consist of a random selection of 500 tweets from major news outlets (e.g., BBC, CNN, Reuters) over the past six months.
Ensuring that the sample is representative is crucial, for example, by balancing sources from different political perspectives. A quantitative content analysis typically allows for a bit of a larger sample because breadth is more important than depth. For a qualitative content analysis, it’s the exact opposite.
Step #3: Defining the Unit of Analysis
At this stage, you specify the level at which your material will be analyzed. For example, if you are studying how climate change is framed in tweets, your unit of analysis could be (1) entire tweets, (2) individual hashtags, or (3) specific phrases related to emotions (e.g., ‘climate crisis’ vs. ‘climate hoax’). If you’re analyzing a text, the unit could be a full sentence or individual words, depending on your research objective.
If you’re looking for semantic nuances, such as emotional tones, it might make sense to analyze individual words. If you’re investigating broader themes, like news “frames,” analyzing entire sentences or text sections may be more appropriate.
Step #4: Defining Descriptive Categories
Before starting the analysis, you need to establish categories for classifying your units of analysis. This involves researching existing coding manuals or codebooks in the academic literature. If none suit your purpose, you must develop your own.
For example, in a study on news framing, a coding manual would list various frame types such as ‘scientific consensus,’ ‘economic impact,’ or ‘conspiracy theory’ and provide instructions for assigning sentences, tweets, videos, or images to these categories.
Authors of coding manuals typically include example cases and detailed coding guidelines, ensuring clarity and consistency. Think of the coding manual as a structured guide for analysis, whether for your use or for others replicating your study.
If you’d like me to create a video on how to develop a coding manual, let me know in the comments!
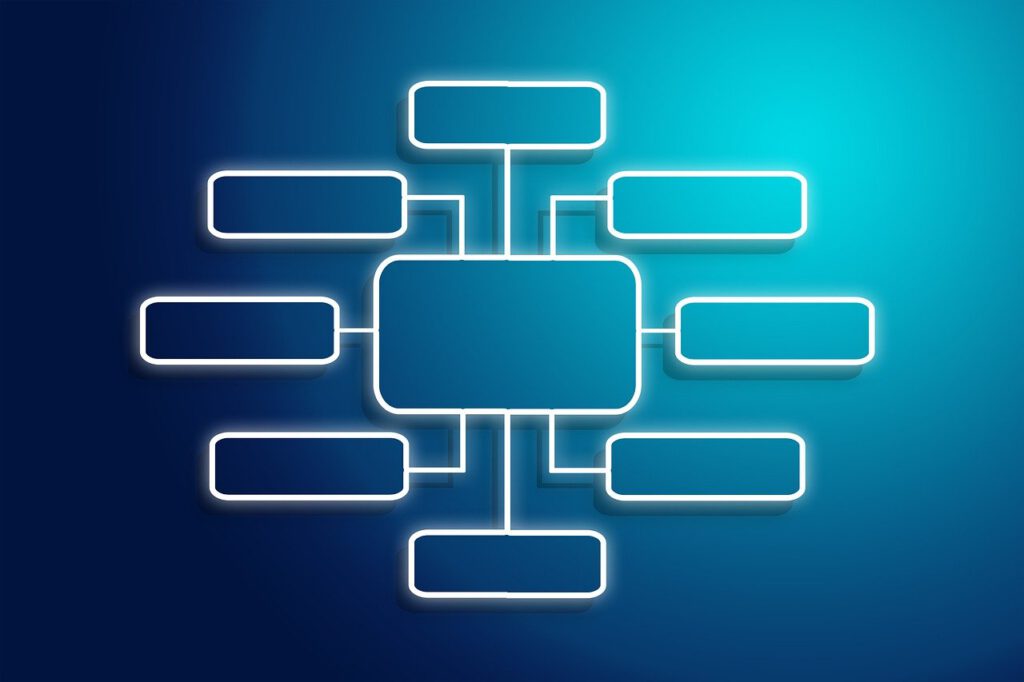
Step #5: Quantification
Once you’ve assigned each unit of analysis to a category, count how often each category appears in your sample. For example, if analyzing 500 tweets, you might find that 40% frame climate change as a ‘scientific consensus,’ while 25% present it as a ‘conspiracy theory.’ These frequencies allow for statistical comparison and further quantitative analysis.
The most common technique for evaluation is frequency analysis, which links category occurrences to the variables under investigation.
According to Krippendorff (1980), key techniques in quantitative content analysis include frequency analysis, contingency analysis, and cluster analysis. He emphasizes that quantitative content analysis must ensure reliability through systematic coding procedures and validation techniques. These methods help uncover statistical patterns while ensuring measurement validity and intercoder reliability.
A crucial aspect of any quantitative content analysis is ensuring reliability and validity. Intercoder reliability should be tested using Krippendorff’s Alpha or Cohen’s Kappa to ensure that different coders classify content consistently. Without strong reliability, the statistical findings of the analysis may not be meaningful.
If you are doing the analysis by yourself, you cannot calculate intercoder reliability. For this case, you may look into “intracoder” reliability.
Step #6: Statistical Analysis
Statistical analysis can be either descriptive (e.g., frequency distributions, cross-tabulations, means, and standard deviations) or inferential, depending on the dataset size. Inferential techniques include regression models to test relationships and factor analyses to identify underlying patterns in large datasets. Descriptive statistics summarize patterns within the data, while inferential techniques, such as regression models, examine relationships between variables. Factor analyses can identify latent patterns in large datasets, while contingency analysis tests the association between different categorical variables. For example, contingency analysis can reveal whether certain frames are more common in specific media sources, while a regression model can test how media framing influences audience perceptions.
For meaningful results, your categories must be clearly operationalized and directly related to the variables under examination. Thus, problem formulation, hypothesis generation, and category selection should be well-aligned.
Step #7: Presenting the Results of Quantitative Content Analysis
When reporting your results, tables are your best friend. First, present the absolute frequencies of your categories and describe them in your own words.
Next, outline the results of your statistical tests, explaining why you chose them and what the findings mean.
Finally, state which of your hypotheses were supported and which were rejected.
Conclusion: Why Choose Quantitative Content Analysis?
Quantitative content analysis is an excellent choice when you want to test an exisintg theory or framework with qualitative data. Some research questions cannot be effectively addressed through traditional quantitative methods like surveys or experiments. In such cases, content analysis provides a valuable alternative.
If this sounds like what you’re looking for—then quantitative content analysis is the right method for you!
Literature on Quantitative Content Analysis
Berelson, B. (1954). Content Analysis. In G. Lindzey (Ed.), Handbook of Social Psychology. Vol. 1: Theory and Method (pp. 488–522). London: Addison-Wesley.
Krippendorff, K. (1980). Content Analysis: An Introduction to Its Methodology. Sage Publications.