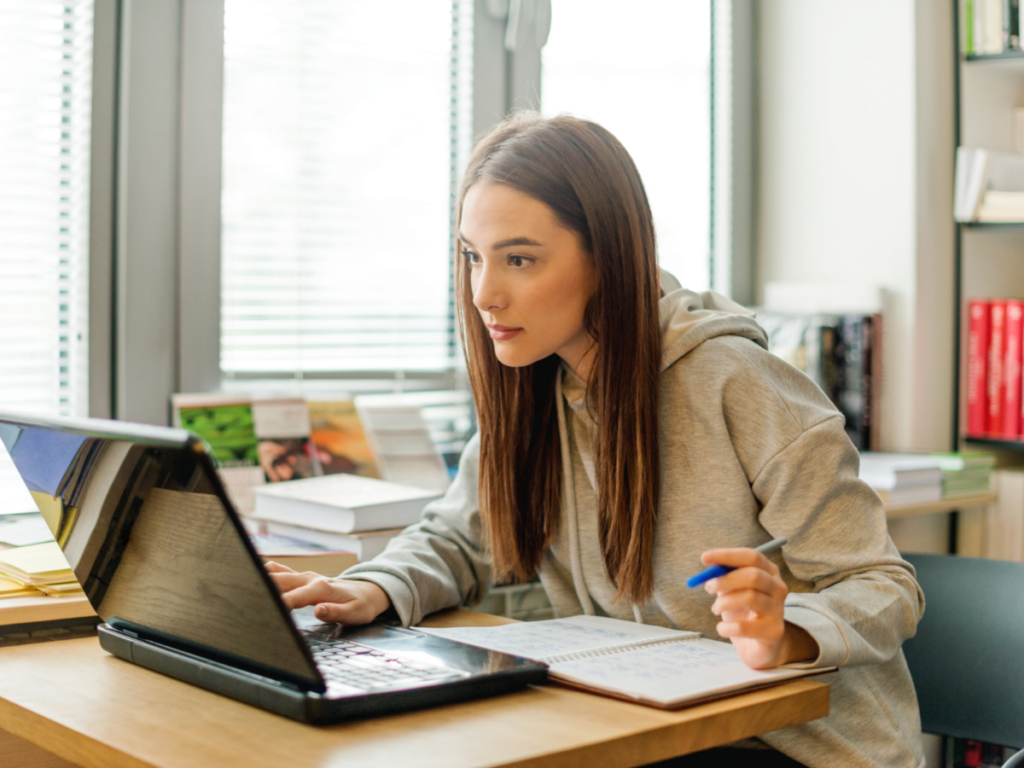
You’ve successfully completed the first step of Grounded Theory, open coding, and now your initial codes are ready. But what’s next? How do you connect these codes meaningfully? That’s exactly where axial coding comes in.
In this video, I’ll show you step-by-step how to apply axial coding, systematically structure your findings, and lay the foundation for your own mini-theory.
#1 What is axial coding?
Axial coding is the second step in the three-stage coding process according to Strauss and Corbin (1998):
- Open coding (data is broken down and labeled as codes)
- Axial coding (relationships between codes are established to form categories)
- Selective coding (core categories are developed)
While open coding breaks down data into smaller thematic units (open codes), axial coding goes further. It helps you discover how these relatively concrete codes relate to each other and how they can be grouped.
This means you’re no longer working directly with your data but rather with the open codes you’ve created. Keep in mind that other Grounded Theory books might use different terminology or slightly altered techniques. Here, when we speak about axial coding, we always refer to the approach of Strauss and Corbin (1998).
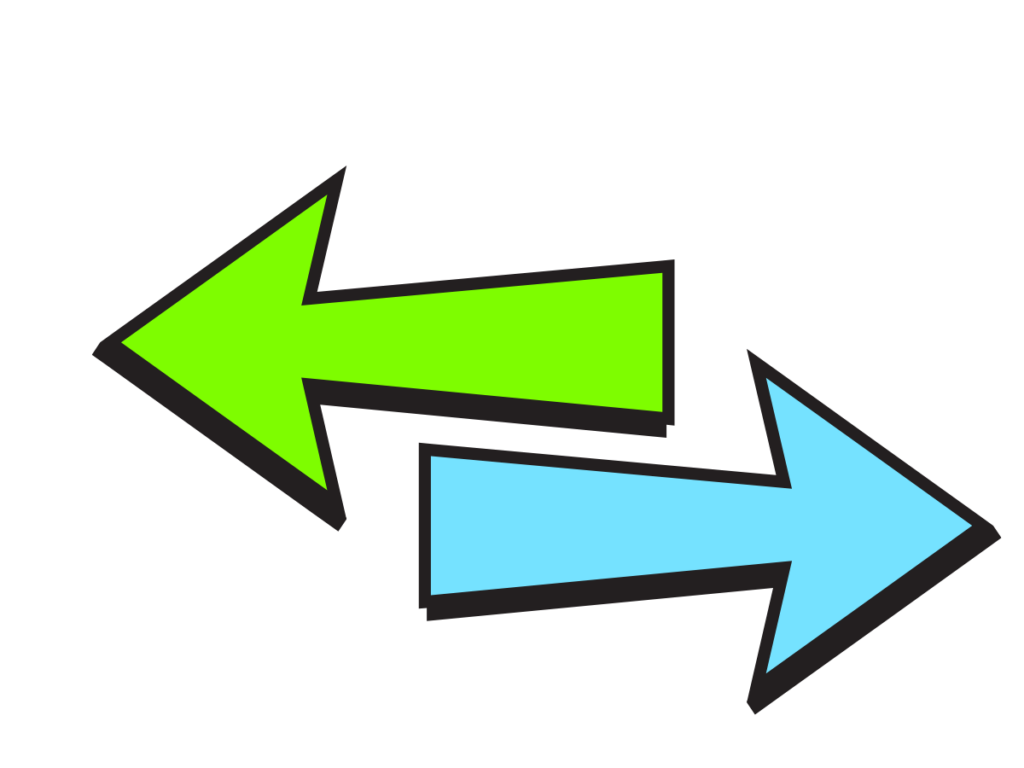
#2 How does axial coding differ from open coding?
A common misconception is that open and axial coding are entirely separate processes. In reality, they often overlap. While creating your initial codes during open coding, you might already notice certain codes seem related.
Axial coding helps systematically explore these relationships. You deliberately ask questions such as:
- Which open codes have a cause-and-effect relationship?
- Are there patterns or regularities in the data?
- How can the open codes be grouped?
Where open coding primarily serves a descriptive function – breaking your data into meaningful units – axial coding has an analytical function. It allows you to reach the next level of abstraction using your open codes.
Example
Imagine you’re studying how students use AI-supported learning programs. Through open coding, you’ve discovered that AI is used in various contexts, like summarizing texts, creating flashcards, or supporting exam preparation.
Here are 6 of your open codes:
- Summarizing lecture scripts
- Creating flashcards
- Audio transcription of lectures
- Live quizzes to revise learning material
- Weighing semester goals
- Creating study plans
But how exactly do students employ AI? Could there be a temporal relationship? This is where axial coding steps in.
You might now group these 6 open codes into logical categories. For instance, “Summarizing lecture scripts” and “Creating flashcards” fit well together under a category called “Preparation.”
“Audio transcription of lectures” and “Live quizzes to revise learning material” might be grouped as “Active Support.”
The remaining two open codes could form a category called “Strategic Planning.”
If you consider the temporal dimension, you might conclude that the categories follow this sequence: (1) Strategic Planning, (2) Preparation, and then (3) Active Support.
This is already a great connection! But wait—is something missing? Do students not use AI for post-learning review? Oops, maybe you didn’t ask this in your interviews! With this realization, you can gather new data to explore exactly that. Perhaps new open codes and a “Review” category will emerge—or maybe not. The point is, after axial coding, you can always return to data collection or open coding. Grounded Theory is iterative, not linear!
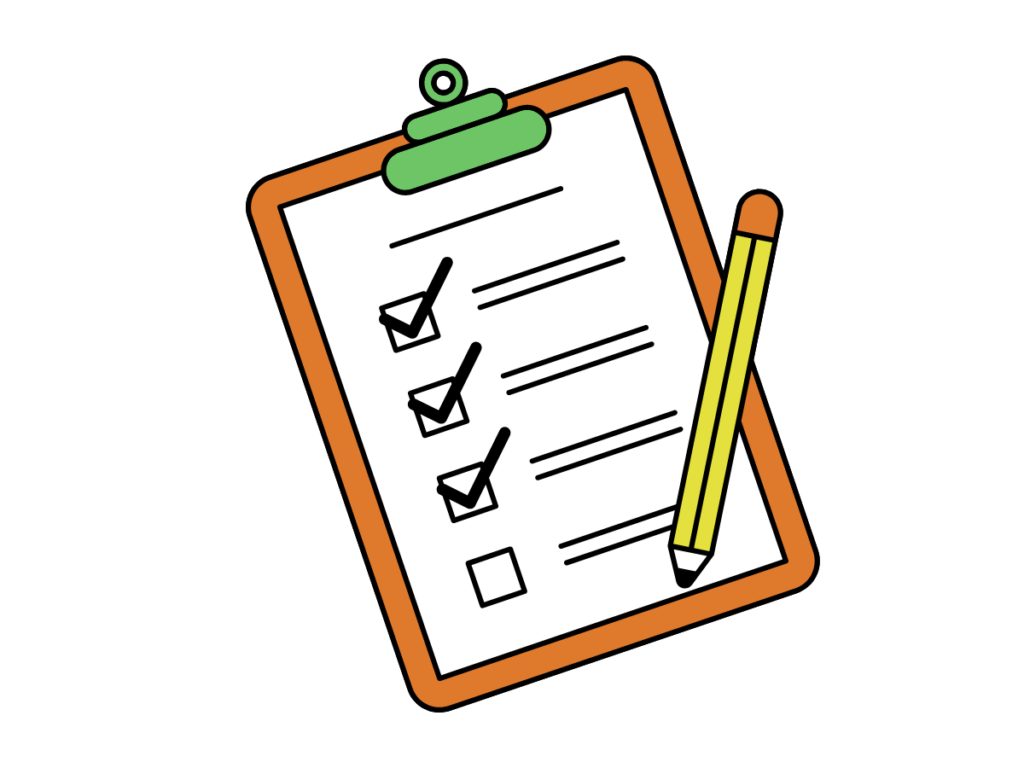
#3 The coding paradigm by Strauss & Corbin (1998)
Strauss and Corbin (1998) offer very concrete guidance. If you’re unsure how to approach axial coding, use their coding paradigm. It helps systematically analyze and structure different aspects of a phenomenon. Let’s clarify this with a practical example.
Imagine you’re analyzing interviews with students about their use of AI tools like ChatGPT for learning. After open coding, you’ve identified several categories, including “Exam preparation,” “Skepticism towards AI,” and “Changes in learning behavior.”
Now you relate these categories to each other. Earlier, we looked at the dimension of time, but there are more aspects. You don’t need to analyze EVERYTHING in the coding paradigm—only what’s necessary for your theory to address your research question.
Phenomenon
The phenomenon describes the central theme or event you’re studying. Example: “Students use AI-supported tools for learning.”
Causal Conditions (Why does it happen?)
These conditions explain what causes or triggers the phenomenon. Example: “Students often have limited time, seeking more efficient learning methods to manage their studies better and cope with exam pressure.”
Context (Under what conditions does the phenomenon occur?)
Context describes specific circumstances or settings where the phenomenon takes place. Example: “In STEM fields such as computer science or engineering, AI usage is more widespread and socially accepted compared to humanities disciplines.”
Intervening Conditions (What additionally influences the phenomenon?)
These factors don’t directly cause but significantly affect how strongly a phenomenon occurs. Example: “Institutional university guidelines, like official prohibitions or recommendations regarding AI use, existing knowledge about AI, or students’ general technical affinity.”
Action Strategies (How do people respond?)
Here you examine how individuals react and what strategies they develop. Example: “Some students use AI tools intensively and regularly, others very selectively or critically reflectively, while still others avoid them completely.”
Consequences (What are the outcomes?)
Consequences describe the outcomes or results of the action strategies applied. Example: “Students alter their learning behavior. This can save time and enhance exam preparation, but might also result in superficial learning or dependency on AI tools.”
#4 Challenges in axial coding
Though axial coding is a powerful technique for theory-building, it can also pose challenges.
Distinguishing between various conditions isn’t always straightforward. Some factors can be interpreted as either causal or intervening conditions. It helps to continuously return to your data, asking: Why is something happening, and what influences it? Also, regularly ask yourself: What exactly should my theory describe? If you’re aiming for a process theory, causal conditions might be less important than mechanisms and temporal progression.
Researchers tend to draw premature conclusions. Once a pattern emerges, you might unintentionally look only for data supporting that pattern. Regularly challenge yourself: Are there alternative explanations? Ideally, use theoretical sampling to collect new data addressing your doubts or gaps in the theory at its current stage.
The coding paradigm should be applied flexibly. Not every study fits perfectly into the provided framework. Some parts may be irrelevant, others crucial. Only you can answer this clearly, keeping your research objective firmly in mind.
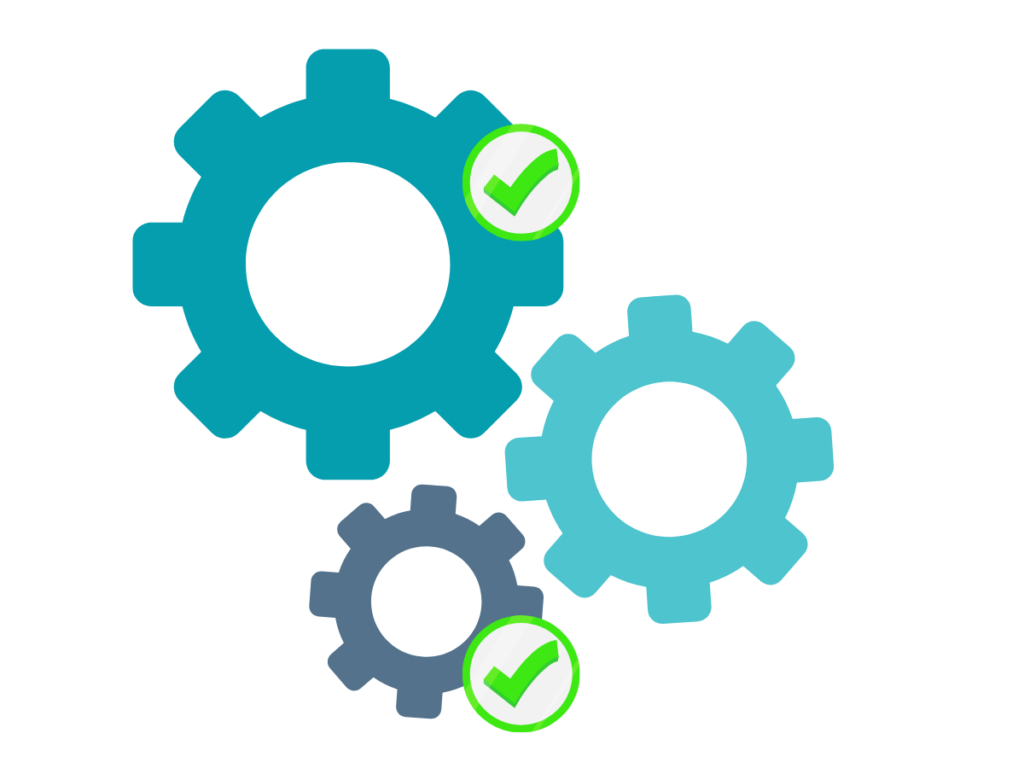
#5 Axial coding as the foundation for theory development
A crucial point in axial coding is that it doesn’t just help structure your data—it also paves the way to theory development.
As you identify more relationships through axial coding, a central category often emerges (sometimes two or three). These categories form the heart of selective coding—the third step of Grounded Theory—where you ascend another level of abstraction.
If, for instance, you find the most critical factor influencing AI use isn’t time pressure but trust in the technology, you might develop a theory of “Technology Acceptance in University Learning.” If you choose this direction, your codes and categories related to time pressure might become less relevant or even discarded in selective coding.
Remember, Grounded Theory is iterative. You’ll likely move back and forth between open and axial coding several times before developing a robust theoretical model.
Conclusion
Axial coding is central to Grounded Theory, enabling you to understand relationships between categories. By systematically applying Strauss and Corbin’s (1998) coding paradigm, you can uncover patterns and connections in your data, laying a solid theoretical foundation.
Once you’ve mastered axial coding, you can tackle selective coding—the final step, where you identify core categories and further develop your theory.
Questions or need help with coding? Drop a comment below!