Qualitative data analysis with ChatGPT is new kid on the block in research nowadays.
This includes thematic analysis, grounded theory, and content analysis. All these methods can benefit from AI.
Because, unfortunately, qualitative data analysis is extremely labor-intensive.
For example, interview transcripts sometimes need to be analyzed sentence by sentence.
Wouldn’t this be a task where you could wonderfully get support from ChatGPT?
Qualitative Data Analysis with ChatGPT
Qualitative data analysis can be used to summarize or structure large amounts of qualitative data, i.e., interview transcripts, documents, or social media postings.
Summarizing means abstracting the content by forming categories, a process also known as “coding.” You assign a “label” to small units of analysis, such as an answer to an interview question, and can thus present hundreds of pages of text in a single category system.
Summarizing also means that you don’t know in advance what categories will emerge. This approach follows an inductive logic, from specific (the data) to general (the categories).
Structuring means assigning the content to pre-defined categories. These categories can, for example, be derived from the literature. This results in a so-called codebook that specifies when an analytical unit corresponds to a certain category.
Both tasks are extremely labor-intensive and repetitive.
That means they are tailor-made for artificial intelligence, or even better, for a language model like GPT.
So, how can we best integrate ChatGPT into the qualitative data analysis process? In the following, we’ll look at 7 steps to turn ChatGPT into your personal research assistant.
The following steps are loosely based on the working paper by Zhang et al. (2023) from Penn State University, where the authors summarized the best practices in prompt engineering from 17 qualitative researchers.
In case you did not know, prompt engineering refers to crafting the instructions you give to ChatGPT and other large language models.
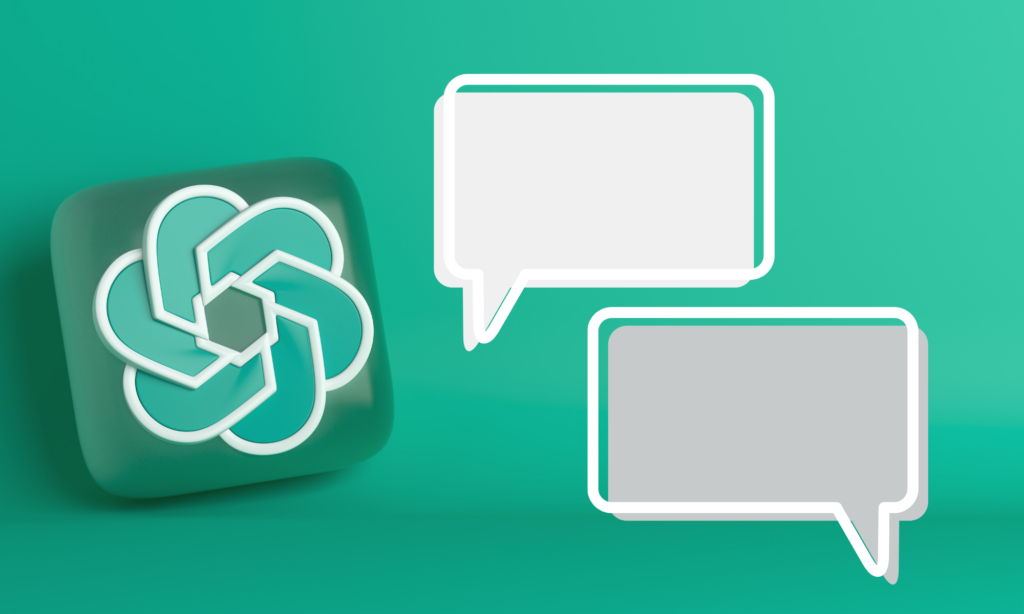
#1 Start your Prompt with a Role-play
Several experiments with ChatGPT have shown that assigning a role can significantly improve the quality of results.
Let’s look at an example.
In our case, ChatGPT is supposed to conduct a thematic analysis with interview data we have collected. So, think of a role that fits this context. For example:
“Imagine you are a researcher and an expert in the evaluation of qualitative data, such as interview transcripts.”
#2 Provide ChatGPT with Context
To perform a qualitative data analysis with ChatGPT, a two-line instruction won’t get you very far. You need to formulate your prompt as detailed as possible to get the best results.
Just imagine you’re talking to someone who is very intelligent but is doing the task for the first time. For our thematic analysis, it could look like this:
“I will provide you with an interview transcript that you should analyze using a method called ‘thematic analysis.’ Your task is to assign categories to the answers in the transcript. A category is an abstract summary of the content. You should not assign categories to the questions themselves.”
#3 Give ChatGPT a Detailed Description of your Qualitative Method
The prompt should describe as precisely as possible what the goal of the task is. This also includes aligning the prompt with the specific goal you have in mind. Let’s say your interviews are about the topic of Remote Work and Stress.
Then you should formulate your prompt accordingly. Always keep in mind that ChatGPT only knows as much about the task as the information you provide to the AI.
“The interview transcripts focus on the topic of remote work. Specifically, the categories you create based on the answers should relate to how remote workers experience stress and what countermeasures they take in this context.”
The level of detail cannot be too high. It’s important not to rush and take your time when composing the prompt.
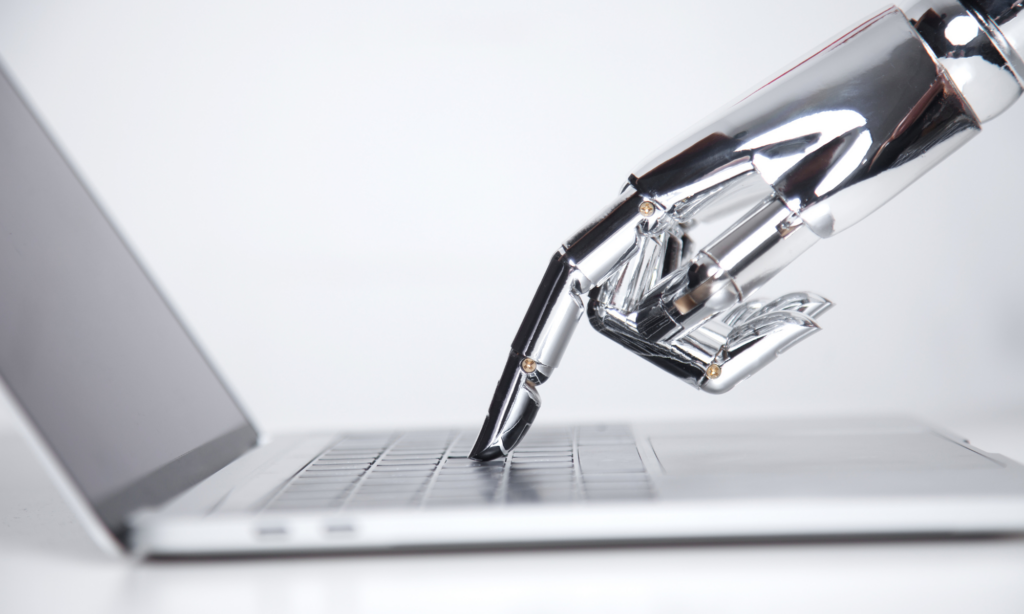
#4 Specify How Results Should Look Like
If you’re working with pre-defined categories or a codebook, now is the time to explain to ChatGPT into which categories the data should be classified. You can also include a complete codebook if you have one.
However, for our example, we’ll continue with an inductive analysis, which means that we develop them from scratch.
Here, we want ChatGPT to keep the categories from being too abstract. I would recommend using the AI only for the labor-intensive tasks, which typically is the first round of coding. This step is often referred to as “open coding”.
Later, when we develop more theoretical codes or themes, we involve our brain a bit more and use our creativity.
For the open coding, we want ChatGPT to give us some examples from the data that represent these categories well.
“Don’t give me more than 10 categories for the first 3 transcripts and, for each category, find a text example of at least three sentences that best reflects the category.”
#5 Structure Your Data
ChatGPT delivers better results when you structure the transcripts.
That means not just copying and pasting but making sure the format of question and answer is consistent.
Spelling errors or punctuation do not affect ChatGPT’s performance.
The easiest way to explain the structure of the transcripts to ChatGPT is as follows:
The transcripts are structured as follows:
<Interviewer (indicated by the abbreviation ‘I’)> : <Question (ending with ‘?’)>
<Participant (indicated by the abbreviation ‘P’)> : <Answer>
#6 Define the Format of the Results
To make it easier for you to process the results and continue to work with them, consider how ChatGPT should present them.
A table would be a good idea in most cases. You could integrate it into your prompt like this:
“Please present the results in table form. The first column contains the categories, the second column contains a description of the categories (at least three sentences), the third column contains the example quote, and the last column contains the number of participants who mentioned the topic.”
#7 Adjust as Needed
After you’ve entered your monster prompt and fed ChatGPT with the transcripts, you can still make adjustments as necessary.
If you feel like the 10 categories are too few, you can ask for more. If you think there are too many, you can instruct ChatGPT to prioritize the categories. For example:
“Reduce the categories to 8 and arrange the table so that the categories mentioned by the most participants are listed first.”
You can then transfer the output table to Excel, for example, and continue working with it.
Qualitative Data Analysis with ChatGPT: Things to Keep in Mind
Are you convinced by the results?
That’s great, but hold on a second. You should be aware of some important limitations of ChatGPT when it comes to qualitative data analysis.
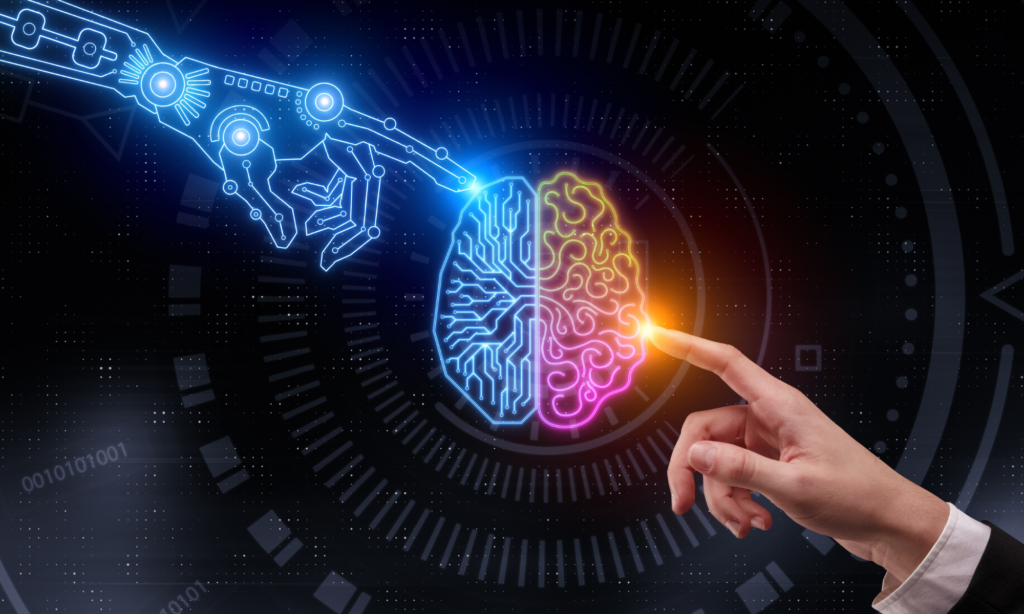
#1 Transparency of Analysis
It’s often challenging to understand how ChatGPT has formed the categories. The authors of the working paper found in their tests that two additional instructions not only improve the results but also enhance transparency.
“Analyze the transcripts sentence by sentence.”
With this instruction, you prompt ChatGPT to analyze the transcripts from beginning to end, just as you would manually. This makes it less likely that ChatGPT skips or neglects parts of the data. So, be sure to include this short sentence.
Another recommendation is to include the following sentence:
“Provide a brief explanation for each category, explaining how you arrived at the category.”
ChatGPT will then provide a description of how the categories relate to the data, making it easier for you to understand how the categories were generated.
#2 ChatGPT Can Get “Tired”
ChatGPT is a black box, meaning we can’t see what’s happening under the hood. If you overburden ChatGPT with many different instructions for an extended period, the quality of results may decline.
The AI is likely to improve over time, and differences between the Pro and free versions may exist, among other factors.
What you should do is create the entire prompt as best as possible and then let ChatGPT process it only once or make only minor adjustments afterwards.
#3 Reliability in Qualitative Data Analysis with ChatGPT
Experiments in which the API of ChatGPT is queried with the same prompts multiple times show that the results are slightly different each time.
This is also known as the “temperature” of the large language model.
The higher the temperature, the more varied the results.
As an average user, you rely on the default settings, where the temperature is not too high. However, what you can (and should) do is perform a manual reliability check, just as you would when coding with another person.
If you’re wondering why I’m not discussing research ethics and plagiarism in this video, simply check out my ChatGPT plagiarism video. There, we cover everything you need to know to use ChatGPT correctly as a tool for your academic projects.