Do you want to analyze qualitative data for your academic work, but the idea of conducting new interviews or collecting documents feels overwhelming? A qualitative meta-study might be the perfect solution for you.
This method has been gaining traction lately. And just like literature reviews, it doesn’t require you to collect your own data…
You can simply use qualitative data from other studies to uncover new connections and develop theories. It doesn’t get much more practical than that, does it?
In this video, I’ll walk you through how to conduct a qualitative meta-study while adhering to academic standards.
1. What is a Qualitative Meta-Study?
A qualitative meta-study (QMS) is a method for combining data from multiple qualitative studies on a specific topic. While a single study often provides only one perspective, QMS allows you to integrate findings from numerous studies, offering a more comprehensive and nuanced understanding.
Meta-analyses are well-established in quantitative research, where they statistically combine numerical data from various studies to draw universal conclusions. In qualitative research, however, the focus is on narrative data, such as interviews and case studies. These are not merely aggregated but reanalyzed, often from a new theoretical perspective, to generate fresh insights.
Until recently, the process for conducting QMS lacked clarity. But in 2024, a groundbreaking paper by Habersang and Reihlen provided detailed guidelines for structuring and standardizing QMS, making the method more accessible and comparable. This paper has been met with widespread acclaim, with many researchers calling it an “instant classic.”
Let’s dive deeper into these guidelines!
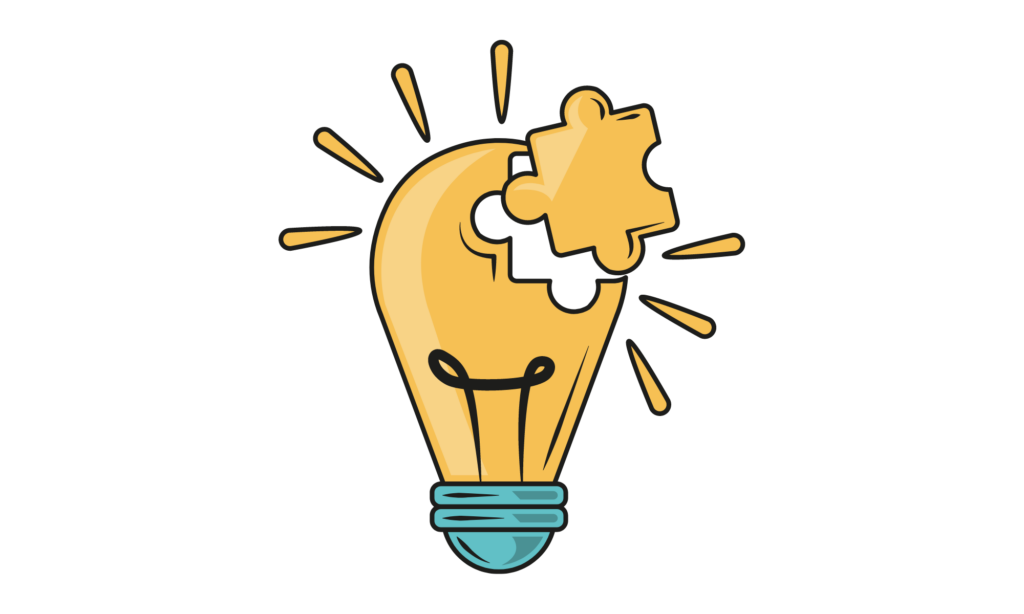
2. The Three Reflective Meta-Practices by Habersang and Reihlen
To ensure qualitative meta-studies yield meaningful insights, Habersang and Reihlen propose three key reflective practices. These practices help you derive deeper understanding from your studies. We’ll cover how to select studies in the next section, but first, here’s what you need to know:
1. Translation
Different studies often use varied terminology for similar concepts. For example, one study might discuss “emotional leadership,” while another refers to “transformational leadership.” Translation involves aligning these terms into a shared language so the studies can be compared effectively.
This process goes beyond mere word alignment—it’s about understanding the underlying meaning of each concept. Your goal is to preserve the essential insights of each study while creating connections between them.
2. Abstraction
Abstraction involves distilling the details of individual studies to identify broader patterns and overarching theories. It’s about lifting the analysis to a higher level, enabling you to see commonalities across studies.
When abstracting, it’s essential to consider the unique context of each study while developing theories that apply across multiple cases. Striking the right balance between detail and generalization is key.
Developing theories might sound intimidating, but it’s not unlike other methods such as grounded theory or theoretical literature reviews. Creating new theoretical insights is often easier than it seems.
3. Iterative Interrogation
Iterative interrogation means revisiting your data repeatedly throughout the analysis to question and refine your assumptions. This process involves continuously challenging your interpretations and adapting them based on new patterns or insights that emerge.
Here, your “data” consists of direct quotes and findings from the qualitative studies you’re analyzing. While you might begin with a specific idea, the iterative process ensures your conclusions evolve as you uncover new evidence.
This constant interplay between critical questioning and discovery helps ensure your research is both innovative and grounded in clear, reproducible results.
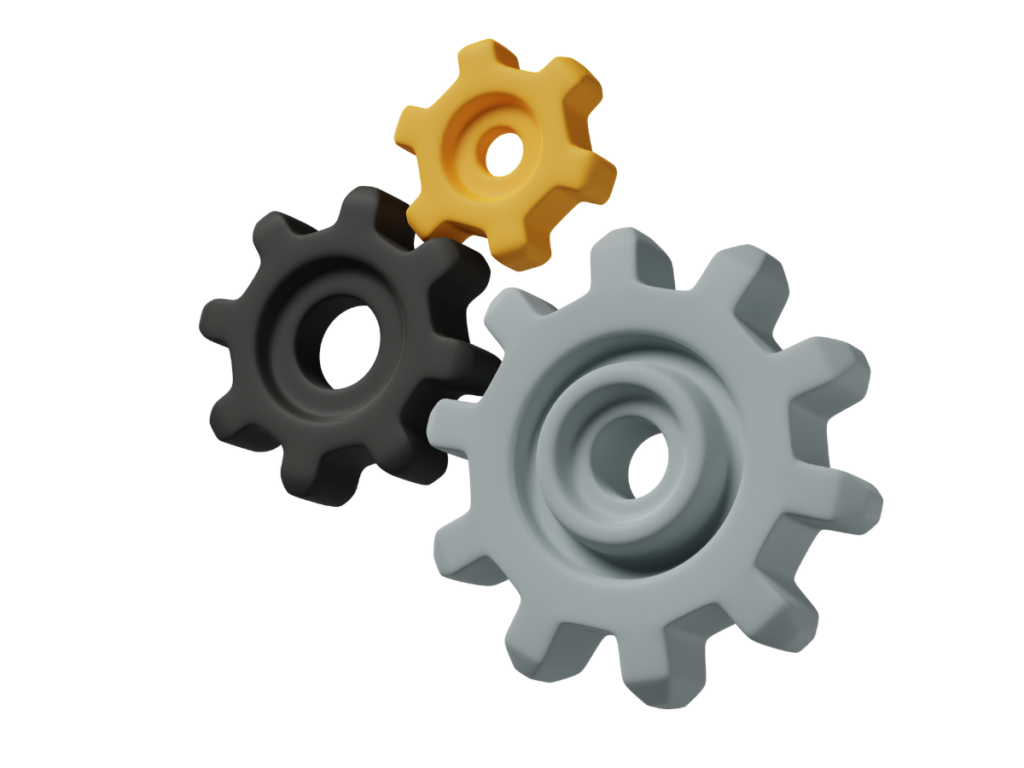
3. Guidelines for Conducting Confirmatory QMS
Confirmatory QMS tests existing theories by comparing findings from multiple studies. The goal is to determine whether the collected data supports or challenges a particular theory. This approach is particularly useful when you want to validate a widely accepted theory or identify inconsistencies across studies.
Guidelines and Procedure:
- Develop a Theory-Driven, Focused Research Question
Start with a precise research question grounded in an existing theory. This question will guide you in formulating specific hypotheses, which you’ll test using data from various studies. Example: “Does transformational leadership increase employee satisfaction in flat hierarchies?” This hypothesis could form the basis for your QMS. - Justify a Comprehensive or Selective Search Strategy
Decide whether to conduct a comprehensive search (aiming to include as many relevant studies as possible) or a selective search (focusing on high-quality studies that align closely with your research question). Example: If you’re studying the impact of transformational leadership in startups, you might specifically look for case studies from that context. - Select Homogeneous and Comparable Cases
Choose cases that are methodologically and theoretically aligned to ensure meaningful comparisons. However, including a few outliers can be useful for testing the boundaries of your theory. Example: If comparing leadership styles, ensure the methods for measuring employee satisfaction are consistent across studies. An outlier might be a study showing that transformational leadership only works in specific cultural contexts. - Synthesize Through Aggregation
Aggregation involves combining the findings of different studies to see whether they support or contradict your hypotheses. Use deductive categories (e.g., “Supports the theory?”) and introduce inductive categories when unexpected patterns arise. The goal is to create a clear theoretical model showing how well your hypotheses hold up. - Ensure Quality Through Transparency
Document every decision and step of your analysis process. Transparency is crucial for ensuring your work can be replicated and validated by others. Maintain a detailed log covering everything from your literature search to your case selection and analysis.
4. Guidelines for Conducting Exploratory QMS
Exploratory QMS focuses on developing new theories or expanding existing ones. The goal is to explore studies for fresh patterns or explanations that might have been overlooked. This method is especially helpful when there are no clear existing theories on the topic.
Guidelines and Procedure:
- Develop an Open Research Question
Keep the research question broad to allow for new ideas and theories to emerge. Refine the question as patterns or insights from the data guide you. Example: You could investigate the phases of digital transformation across organizations without relying on a predefined theoretical framework. - Broad or Targeted Literature Search
Conduct a broad search to capture diverse data or focus on particularly rich studies that provide deep insights into specific aspects of your topic. Example: Collect case studies from various industries to understand how digital transformation unfolds in different settings. - Choose Heterogeneous and Diverse Cases
Include diverse and contrasting cases to uncover new perspectives and patterns that might not emerge in a homogeneous dataset. - Synthesize Through Configuration
Reinterpret the data creatively to develop a new theoretical model, rather than forcing it into predefined categories. Goal: Generate fresh insights about the phenomenon by integrating findings from different studies. - Ensure Quality Through Diversity and Depth
The success of exploratory QMS depends on the variety and depth of the analyzed cases. The better you identify and articulate new patterns, the stronger your theoretical contribution.
Summary of QMS Types
Here’s a quick comparison of the two types of QMS:
Criterion | Confirmatory QMS | Exploratory QMS |
---|---|---|
Goal | Test and refine existing theories | Develop new theories |
Research Question | Focused, theory-driven | Open, broadly defined |
Hypotheses | Predefined | None—focused on discoveries |
Search Strategy | Comprehensive or selective | Broad, but targeted cases also possible |
Sample | Homogeneous and comparable | Heterogeneous and diverse |
Synthesis | Aggregation of findings | Configuration of new theoretical models |
Quality Criteria | Testing and refining theories | Developing new theories |
A qualitative meta-study is certainly not the easiest method to tackle, but it’s a perfectly feasible choice for something like a master’s thesis. If you already have experience with qualitative research or are willing to invest the time to learn this method, suggesting a QMS can really impress your supervisors.
Good luck with your research!
📖 Habersang, S., & Reihlen, M. (2024). Advancing qualitative meta-studies (QMS): Current practices and reflective guidelines for synthesizing qualitative research. Organizational Research Methods.