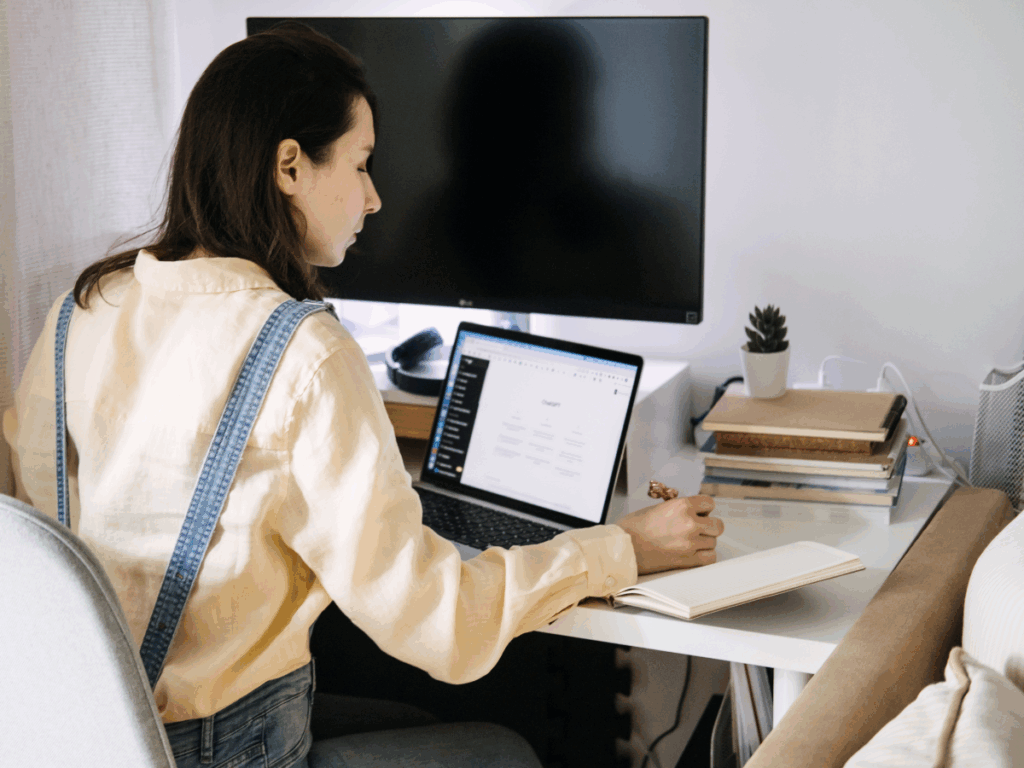
Learn how to use ChatGPT for Academic Writing – if you’ve ever used AI for academic writing, you’re probably familiar with the dilemma: where’s the line between smart support and academic misconduct?
Plagiarism can have serious consequences – ranging from poor grades to having your entire paper invalidated. But does that mean you shouldn’t use ChatGPT at all? Not at all! In fact, there are many smart and ethical ways to integrate AI into your academic writing process without falling into the plagiarism trap. And that’s exactly what this post is about!
Today, I’ll walk you through seven ways to use ChatGPT effectively and ethically in your academic writing.
1. Identifying Research Gaps and Formulating Hypotheses
You’ve done your research, but it feels like everything has already been said about your topic? ChatGPT can help you uncover unanswered research questions or methodological weaknesses in existing studies. This technique is used by many researchers to develop innovative research ideas – a real advantage if you’re writing your Bachelor’s or Master’s thesis.
ChatGPT is well-known for its ability to generate creative ideas. According to a study by Lee and Chung (2024), ChatGPT outperforms traditional tech-based brainstorming methods – like Googling – by a wide margin. This is because the AI can combine seemingly unrelated concepts in novel ways, sparking new insights.
Another trick: Use ChatGPT as a peer reviewer for your ideas. Ask it to evaluate your research question from the perspective of a critical academic. This can give you valuable insights on where to refine your approach.
Once you’ve got your research question, you can also use ChatGPT to support the hypothesis-building phase. Rather than asking it to generate a ready-made hypothesis, use it to explore relevant variables or test different hypothesis formats. For example, if your topic is the impact of remote work on productivity, you might ask: “What factors influence productivity when working from home?” or “Are there studies showing a positive correlation between remote work and increased productivity? What were the methodological, theoretical, or conceptual limitations of those studies?”
Try to think a little outside the box when writing your prompts – instead of just asking it to generate this or that.
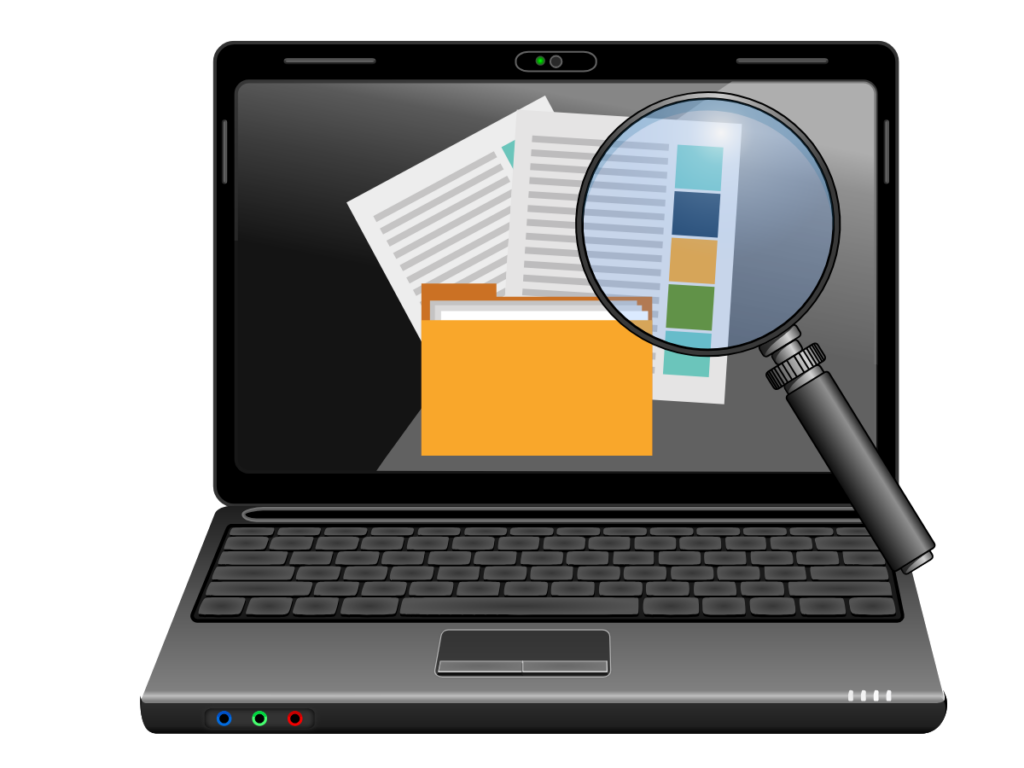
2. Conducting a More Targeted Literature Search
Many students spend hours on Google Scholar or other academic databases without a clear system or search strategy. This typically leads to two major problems: First, relevant studies are often missed because the search terms aren’t well chosen. Second, the overwhelming number of search results can make it difficult to identify the most important and high-quality sources.
Instead of just typing keywords into a search bar, ask ChatGPT for support: Request relevant key terms or alternative expressions that are commonly used in academic literature. For instance, the term “digital learning” might also appear in studies as “e-learning” or “computer-assisted learning”. By asking ChatGPT for these synonyms, you’re less likely to miss key studies.
To narrow down the flood of results, ask ChatGPT which journals are the most reputable in your field. This will help you target your search and find higher-quality sources. Professors want to see papers that cite studies from the journals and conferences they themselves read and publish in. The quality of your references matters more than the topic match. Imagine you find a study that fits your research topic perfectly. If it’s from an obscure journal, it’s practically irrelevant to your academic field. It sounds harsh, but that’s the reality. Your reference list will be judged based on the quality of the sources it includes. So always make sure your argumentation is grounded in the most respected research.
However, having ChatGPT search for studies for you? That’s something you should seriously reconsider. In a study published in the prestigious PNAS journal, Lehr et al. (2024) tested ChatGPT’s performance across various research-related tasks. Literature searching was where the AI performed the worst. Interestingly, it did quite well when it came to advising on research ethics – which is kind of funny, if you think about it.
3. Improving Your Methodology
Choosing the right research method is one of the biggest challenges in academic writing. Instead of being unsure whether to go with a literature-based approach, or a qualitative or quantitative design, you can ask ChatGPT: “Which empirical method is best suited to answer my research question?” This can help you weigh the pros and cons of different methods more effectively.
Ideally, you choose the method that best fits your research question. But in reality, it’s often the other way around. Your supervisor tells you, “Please conduct interviews.” And now the method is fixed – your task becomes finding a research question that fits the method.
Operationalizing your concepts is also essential. ChatGPT can help you identify suitable items or scales that have already been used in previous studies. That way, you ensure your variables are clearly defined and measurable.
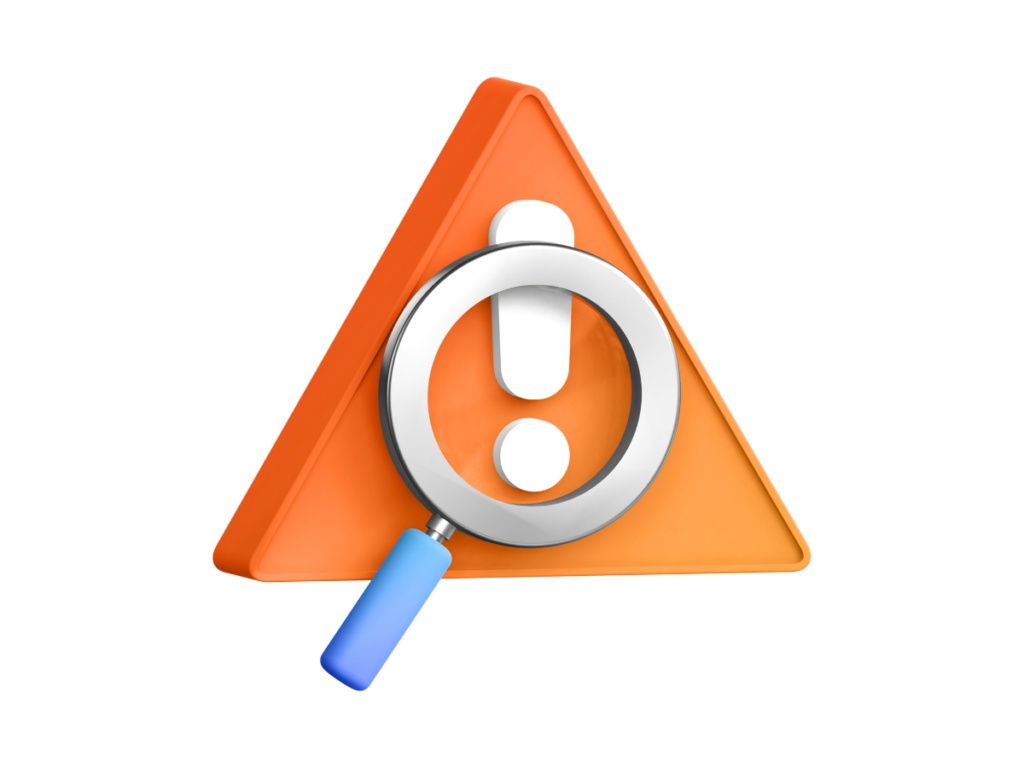
You can also use the AI to critically reflect on your methodology. Ask: “What methodological weaknesses might arise in my study?” This will help you identify potential limitations early on – and address them proactively.
If you’re planning an empirical study, ChatGPT can also assist in selecting appropriate statistical techniques or methods of analysis. This ensures that your data is analyzed systematically and with solid reasoning. However, you should not let ChatGPT analyze your data directly – the risk of errors is still too high. Instead, perform your statistical tests yourself and use ChatGPT to review your approach. According to Lehr et al. (2024), ChatGPT is quite good at spotting when you’re not following statistical best practices.
4. Researching Theories
Theories are the backbone of any academic paper. ChatGPT can give you an initial orientation by suggesting theories that might be relevant to your topic. Important: AI is not an academic source! Use ChatGPT as a starting point to explore theoretical frameworks, and once you’ve identified one that fits, switch to scholarly literature for further research.
A clever strategy is to ask ChatGPT to compare different theories, for example: “Compare Theory X with Theory Y – what are their strengths and weaknesses in relation to Topic X?” This kind of overview can make the decision-making process during your “theory casting” much easier.
You can also ask ChatGPT to name specific applications or research fields where a given theory is frequently used. This helps you assess how relevant a theory might be for your project — or, conversely, highlight that a theory has already been thoroughly examined in a specific area.
Another smart approach is to ask the AI about theoretical developments or critical debates: “How has Theory X evolved over the past ten years?” or “What are the main criticisms of Theory Y?” This gives you not just a general overview, but also insight into which aspects of a theory are currently debated or evolving.
Additionally, ChatGPT can help you draw interdisciplinary connections: “Are there overlaps between Theory X from psychology and Theory Y from sociology?” This can help you uncover new perspectives for your research and possibly create original theoretical links in your paper.
5. Strengthening Your Argument
A strong academic paper relies on clear argumentation and thoughtful discussion. And this is where ChatGPT can help in a somewhat unconventional way. Imagine you’ve constructed an argument that seems perfectly logical and convincing to you — but how would a critical reviewer assess it?
Most students overlook potential weaknesses in their arguments. But you can ask ChatGPT to deliberately deconstruct your reasoning. For example, ask: “What arguments could be used to contradict my thesis?” or “How might a critic challenge my reasoning?”
This turns ChatGPT into a “devil’s advocate,” giving you a fresh perspective on your work. It also allows you to proactively incorporate counterarguments — making your argumentation more robust and resistant to criticism.
6. Ensuring Argumentative Consistency
Once you’ve found strong arguments, it’s important to maintain consistency throughout your paper. Especially in longer texts, contradictions or unclear transitions can easily sneak in.
ChatGPT can help identify these inconsistencies if you ask it to systematically analyze your argument and point out any logical breaks. Try prompts like: “Identify inconsistencies or flawed reasoning in my text.”
Another common issue in academic writing is a lack of clear logical structure. Transitions between arguments might be missing, or there may be logical jumps that are hard for the reader to follow. ChatGPT can help by checking your paper for coherence and suggesting ways to improve the flow between points. A simple prompt would be: “Do you notice any logical leaps or missing links between my arguments?”
For smoother transitions, try: “How can I improve the argumentative connection between Section A and Section B?”
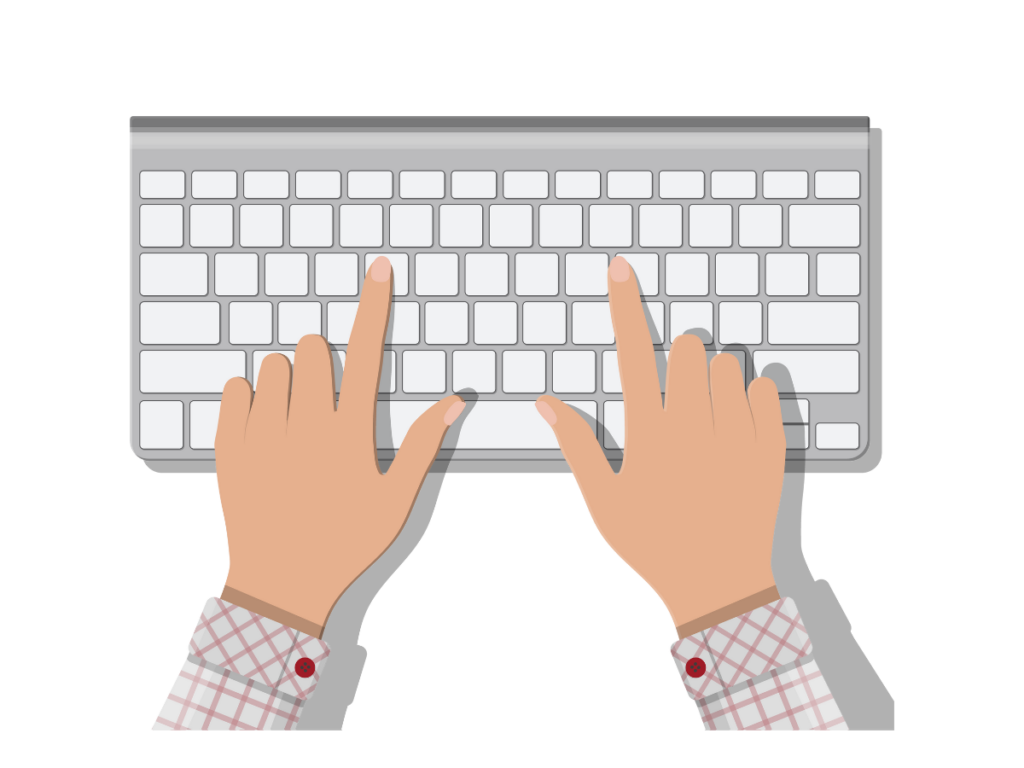
7. Improving Academic Writing Style
Another challenge many students face is mastering the academic writing style, and with ChatGPT, many simply ask the tool to rephrase their text to make it sound “better.”
But here’s what most don’t realize: ChatGPT can also help you improve your academic writing more deliberately. Instead of just asking it to rephrase your text, have it analyze and critique your writing style. For instance, you could ask: “What common writing mistakes do students in my field make?” or “Is my writing style appropriate for an academic paper?”
Keep in mind that ChatGPT has been trained on thousands of academic articles. So what it considers “academic” often reflects phrasing that frequently appears in published studies. This has led to a trend where professors joke about texts that overuse words like “crucial” or “key.” The widespread use of ChatGPT has caused an inflation of these terms. Don’t fall into this trap — and don’t let the AI drain the originality from your writing style.
One especially useful feature is that you can ask ChatGPT to adapt your style to a specific journal. If you’re planning to submit your paper to a respected journal, you can ask the AI to revise your text to match the style typically used in that publication. This helps you understand what academic writing looks like across different disciplines.
Final Thoughts – ChatGPT for Academic Writing
ChatGPT can make academic writing easier — but it’s no substitute for your own analysis and critical thinking. If used wisely, it can help you build better structures, sharpen your research question, compare theories, strengthen your arguments, and refine your writing style.
But don’t use ChatGPT to generate entire sections of your paper or accept its arguments uncritically. And above all: don’t use AI-generated citations! ChatGPT often invents sources — and that can lead to serious consequences. If you’re using an AI tool for literature searches, make sure it provides a link to the original source.
Never rely blindly on AI. Always verify academic sources yourself and treat ChatGPT as a research assistant — not a replacement for you as a researcher.
References
Lee, B.C., Chung, J.(. An empirical investigation of the impact of ChatGPT on creativity. Nat Hum Behav 8, 1906–1914 (2024). https://doi.org/10.1038/s41562-024-01953-1
Lehr, S. A., Caliskan, A., Liyanage, S., & Banaji, M. R. (2024). ChatGPT as research scientist: probing GPT’s capabilities as a research librarian, research ethicist, data generator, and data predictor. Proceedings of the National Academy of Sciences, 121(35), 1-9. https://doi.org/10.1073/pnas.2404328121